Tips on how to use earthquake information to mannequin boundaries is a vital side of understanding and mapping tectonic plate interactions. This information offers a complete overview of using earthquake information, from its various sorts and traits to stylish modeling methods and information integration methods. The evaluation of earthquake information permits for the identification of boundaries, the prediction of seismic exercise, and a deeper understanding of the dynamic Earth.
The preliminary levels contain understanding the assorted kinds of earthquake information related to boundary modeling, together with magnitude, location, depth, and focal mechanisms. Subsequently, the info is preprocessed to deal with points resembling lacking values and outliers. This refined information is then utilized in geospatial modeling methods, resembling spatial evaluation, to determine patterns and anomalies, enabling the identification of plate boundaries.
Integrating earthquake information with different geological information sources, like GPS information and geophysical observations, enhances the mannequin’s accuracy and reliability. The ultimate levels contain evaluating the mannequin’s accuracy, speaking the outcomes by way of visible aids, and sharing insights with the scientific group.
Introduction to Earthquake Information for Boundary Modeling
Earthquake information offers essential insights into the dynamic nature of tectonic plate boundaries. Understanding the patterns and traits of those occasions is crucial for growing correct fashions of those complicated methods. This information encompasses a variety of data, from the exact location and magnitude of an earthquake to the intricate particulars of its supply mechanism.Earthquake information, when analyzed comprehensively, permits for the identification of stress regimes, fault orientations, and the general motion of tectonic plates.
This, in flip, facilitates the event of fashions that precisely depict plate interactions and potential future seismic exercise.
Earthquake Information Varieties Related to Boundary Modeling
Earthquake information is available in numerous varieties, every contributing to a complete understanding of plate interactions. Key information sorts embody magnitude, location, depth, and focal mechanism. These traits, when analyzed collectively, reveal crucial details about the earthquake’s supply and its implications for boundary modeling.
Traits of Earthquake Datasets
Totally different datasets seize distinct elements of an earthquake. Magnitude quantifies the earthquake’s power launch. The placement pinpoints the epicenter, the purpose on the Earth’s floor straight above the hypocenter (the purpose of rupture). Depth measures the gap from the floor to the hypocenter, whereas the focal mechanism reveals the orientation and motion of the fault aircraft in the course of the rupture.
Significance of Earthquake Information in Understanding Tectonic Plate Boundaries
Earthquake information performs a pivotal position in understanding tectonic plate boundaries. The distribution of earthquakes throughout the globe displays the relative movement and interplay between plates. Concentrations of seismic exercise usually delineate plate boundaries, resembling convergent, divergent, and rework boundaries.
Relationship Between Earthquake Occurrences and Plate Interactions
Earthquake occurrences are strongly correlated with plate interactions. At convergent boundaries, the place plates collide, earthquakes are sometimes deeper and extra highly effective. Divergent boundaries, the place plates transfer aside, exhibit shallower earthquakes. Rework boundaries, the place plates slide previous one another, generate a spread of earthquake magnitudes and depths.
Abstract of Earthquake Information Varieties and Purposes
Information Sort | Measurement | Unit | Software in Boundary Modeling |
---|---|---|---|
Magnitude | Vitality launched | Richter scale, Second magnitude | Assessing earthquake power and potential affect, figuring out areas in danger. |
Location | Epicenter coordinates | Latitude, Longitude | Defining the spatial distribution of earthquakes, mapping energetic fault zones. |
Depth | Distance from floor to hypocenter | Kilometers | Characterizing the kind of plate boundary (e.g., shallow at divergent boundaries, deeper at convergent). |
Focal Mechanism | Fault aircraft orientation and motion | Strike, dip, rake | Figuring out the course of plate movement, figuring out the stress regime, and predicting future earthquake places. |
Information Preprocessing and Cleansing
Earthquake datasets usually comprise inconsistencies and inaccuracies, making them unsuitable for direct use in boundary modeling. These points can vary from lacking location information to inaccurate magnitudes. Strong preprocessing is essential to make sure the reliability and accuracy of the next evaluation. Addressing these points enhances the standard and reliability of the outcomes obtained from the mannequin.
Widespread Information High quality Points in Earthquake Datasets
Earthquake information can undergo from numerous high quality points. Incomplete or lacking info, like lacking depth or location coordinates, is frequent. Inconsistent items or codecs, like completely different magnitude scales used throughout numerous datasets, will also be problematic. Outliers, representing uncommon or inaccurate readings, can considerably skew the mannequin’s outcomes. Incorrect or inconsistent metadata, resembling reporting errors or typos, may compromise the integrity of the dataset.
Information entry errors are a significant concern.
Dealing with Lacking Values
Lacking values in earthquake information are sometimes dealt with by way of imputation. Easy strategies embody utilizing the imply or median of the present values for a similar variable. Extra subtle methods, like utilizing regression fashions or k-nearest neighbors, can predict lacking values primarily based on associated information factors. The collection of the imputation methodology will depend on the character of the lacking information and the traits of the dataset.
It is essential to doc the imputation methodology used to keep up transparency.
Dealing with Outliers
Outliers in earthquake datasets can come up from numerous sources, together with measurement errors or uncommon occasions. Detecting and dealing with outliers is crucial to make sure the accuracy of boundary modeling. Statistical strategies just like the interquartile vary (IQR) or the Z-score can be utilized to determine outliers. As soon as recognized, outliers may be eliminated, changed with imputed values, or handled as separate instances for additional evaluation.
The choice on find out how to deal with outliers ought to take into account the potential affect on the modeling outcomes and the character of the outliers themselves.
Information Normalization and Standardization
Normalizing and standardizing earthquake information is crucial for a lot of modeling duties. Normalization scales the info to a particular vary, usually between 0 and 1. Standardization, alternatively, transforms the info to have a imply of 0 and a regular deviation of 1. These methods can enhance the efficiency of machine studying algorithms by stopping options with bigger values from dominating the mannequin.
For instance, earthquake magnitudes would possibly must be normalized if different variables have a lot smaller values.
Structured Strategy to Information Filtering and Cleansing
A structured method is crucial for effectively cleansing and filtering earthquake information. This includes defining clear standards for filtering and cleansing, and implementing constant procedures to handle lacking values, outliers, and inconsistent information. Clear documentation of the steps taken is crucial for reproducibility and understanding the modifications made to the dataset.
Desk of Preprocessing Steps
Step | Description | Technique | Rationale |
---|---|---|---|
Determine Lacking Values | Find situations the place information is absent. | Information inspection, statistical evaluation | Important for understanding information gaps and guiding imputation methods. |
Impute Lacking Values | Estimate lacking values utilizing acceptable strategies. | Imply/Median imputation, regression imputation | Change lacking information with believable estimates, avoiding full removing of knowledge factors. |
Detect Outliers | Determine information factors considerably deviating from the norm. | Field plots, Z-score evaluation | Helps pinpoint and deal with information factors doubtlessly resulting in inaccurate modeling outcomes. |
Normalize Information | Scale values to a particular vary. | Min-Max normalization | Ensures that options with bigger values don’t unduly affect the mannequin. |
Standardize Information | Rework values to have a imply of 0 and commonplace deviation of 1. | Z-score standardization | Permits algorithms to match information throughout completely different items or scales successfully. |
Modeling Methods for Boundary Identification
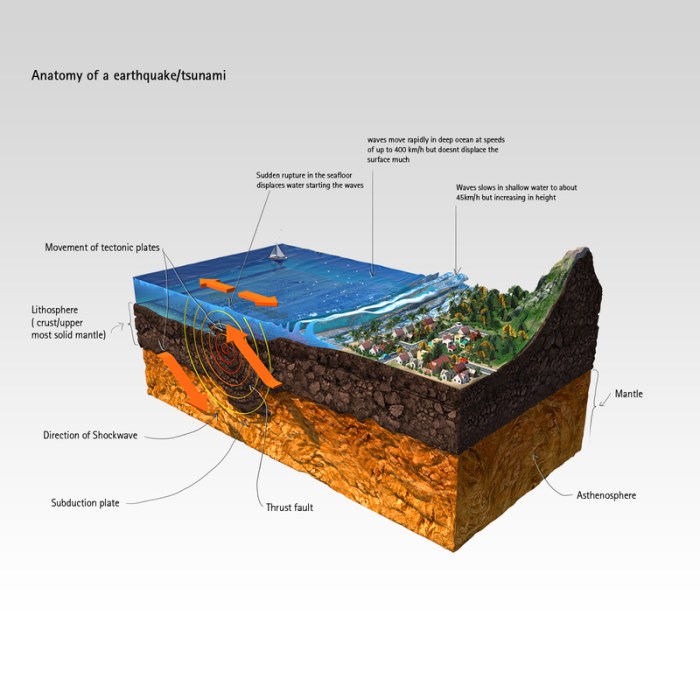
Earthquake information, when correctly analyzed, can reveal essential insights into the dynamic nature of tectonic boundaries. Understanding the spatial distribution, frequency, and depth of earthquakes permits us to mannequin these boundaries and doubtlessly predict future seismic exercise. This understanding is essential for mitigating the devastating affect of earthquakes on susceptible areas.Numerous geospatial and statistical modeling methods may be utilized to earthquake information to determine patterns, anomalies, and potential future seismic exercise.
These methods vary from easy spatial evaluation to complicated statistical fashions, every with its personal strengths and limitations. A crucial analysis of those methods is crucial for choosing essentially the most acceptable methodology for a given dataset and analysis query.
Geospatial Modeling Methods
Spatial evaluation instruments are basic to exploring patterns in earthquake information. These instruments can determine clusters of earthquakes, delineate areas of excessive seismic exercise, and spotlight potential fault traces. Geospatial evaluation allows the visualization of earthquake occurrences, permitting researchers to rapidly grasp the spatial distribution and potential correlations with geological options. This visible illustration can reveal anomalies that may not be obvious from tabular information alone.
Statistical Strategies for Earthquake Clustering and Distribution
Statistical strategies play a crucial position in quantifying the spatial distribution and clustering of earthquakes. These strategies assist to find out whether or not noticed clusters are statistically important or merely random occurrences. Methods resembling level sample evaluation and spatial autocorrelation evaluation may be employed to evaluate the spatial distribution of earthquake occurrences and determine areas of upper likelihood of future seismic occasions.
These statistical measures present quantitative proof supporting the identification of potential boundaries.
Predicting Future Seismic Exercise and its Affect on Boundaries
Predicting future seismic exercise is a fancy problem, however modeling methods can be utilized to evaluate the potential affect on boundaries. Historic earthquake information can be utilized to determine patterns and correlations between seismic occasions and boundary actions. Refined fashions, incorporating numerous components like stress buildup, fault slip charges, and geological circumstances, may also help assess the chance of future earthquakes and estimate their potential affect.
As an illustration, simulations can predict the displacement of boundaries and the resultant results, resembling floor deformation or landslides. The 2011 Tohoku earthquake in Japan, the place exact measurements of displacement have been recorded, highlights the significance of those predictions in understanding the dynamic habits of tectonic plates.
Comparability of Modeling Methods
Approach | Description | Strengths | Limitations |
---|---|---|---|
Spatial Autocorrelation Evaluation | Quantifies the diploma of spatial dependence between earthquake places. | Identifies areas of excessive focus and potential fault zones. Gives a quantitative measure of spatial clustering. | Assumes a stationary course of; could not seize complicated spatial relationships. May be computationally intensive for giant datasets. |
Level Sample Evaluation | Examines the spatial distribution of earthquake epicenters. | Helpful for figuring out clusters, randomness, and regularity in earthquake distributions. | May be delicate to the selection of research window and the definition of “cluster.” Could not all the time straight pinpoint boundary places. |
Geostatistical Modeling | Makes use of statistical strategies to estimate the spatial variability of earthquake parameters. | Can mannequin spatial uncertainty in earthquake location and magnitude. | Requires important information and experience to construct and interpret fashions. Is probably not appropriate for complicated geological settings. |
Machine Studying Algorithms (e.g., Neural Networks) | Make use of complicated algorithms to determine patterns and predict future occasions. | Excessive potential for predictive energy; can deal with complicated relationships. | May be “black field” fashions, making it obscure the underlying mechanisms. Require massive datasets for coaching and will not generalize properly to new areas. |
Spatial Evaluation of Earthquake Information
Understanding earthquake information requires contemplating its geographical context. Earthquake occurrences usually are not random; they’re usually clustered in particular areas and alongside geological options. This spatial distribution offers essential insights into tectonic plate boundaries and the underlying geological constructions liable for seismic exercise. Analyzing this spatial distribution helps delineate the boundaries and determine patterns that is perhaps missed by purely statistical evaluation.
Geographical Context in Earthquake Information Interpretation
Earthquake information, when considered by way of a geographical lens, reveals important patterns. For instance, earthquakes ceaselessly cluster alongside fault traces, indicating the situation of energetic tectonic boundaries. The proximity of earthquakes to recognized geological options, resembling mountain ranges or volcanic zones, can recommend relationships between seismic exercise and these options. Analyzing the spatial distribution of earthquakes, subsequently, offers crucial context for decoding the info, revealing underlying geological processes and figuring out areas of potential seismic danger.
Earthquake Information Visualization
Visualizing earthquake information utilizing maps and geospatial instruments is crucial for understanding spatial patterns. Numerous mapping instruments, resembling Google Earth, ArcGIS, and QGIS, enable overlaying earthquake epicenters on geological maps, fault traces, and topographic options. This visible illustration facilitates the identification of spatial relationships and clusters, offering a transparent image of earthquake distribution. Moreover, interactive maps allow customers to zoom in on particular areas and look at the main points of earthquake occurrences, permitting a deeper understanding of the info.
Coloration-coded maps can spotlight the depth or magnitude of earthquakes, emphasizing areas of upper seismic danger.
Spatial Autocorrelation in Earthquake Incidence
Spatial autocorrelation evaluation quantifies the diploma of spatial dependence in earthquake occurrences. Excessive spatial autocorrelation means that earthquakes are likely to cluster in sure areas, whereas low spatial autocorrelation implies a extra random distribution. This evaluation is essential for figuring out patterns and clusters, which may then be used to outline and refine boundary fashions. Software program instruments carry out this evaluation by calculating correlations between earthquake occurrences at completely different places.
The outcomes of this evaluation can then be used to determine areas the place earthquake clusters are prone to happen.
Earthquake Distribution Throughout Geographic Areas
Analyzing the distribution of earthquakes throughout completely different geographic areas is significant for understanding regional seismic hazards. Totally different areas exhibit completely different patterns of earthquake exercise, that are straight linked to the underlying tectonic plate actions. Comparative evaluation of those patterns helps delineate the boundaries of those areas and their relative seismic exercise. For instance, the Pacific Ring of Hearth is a area of excessive seismic exercise, exhibiting a definite sample of clustered earthquake occurrences.
Geospatial Instruments for Earthquake Boundary Evaluation
Numerous geospatial instruments supply particular functionalities for analyzing earthquake information. These instruments facilitate the identification of boundaries and supply insights into spatial patterns in earthquake occurrences.
- Geographic Data Methods (GIS): GIS software program like ArcGIS and QGIS enable for the creation of maps, the overlay of various datasets (e.g., earthquake information, geological maps), and the evaluation of spatial relationships. GIS can deal with massive datasets, and its capabilities make it an indispensable software in boundary delineation from earthquake information.
- World Earthquake Mannequin Databases: Databases such because the USGS earthquake catalog present complete info on earthquake occurrences, together with location, time, magnitude, and depth. These databases are invaluable sources for analyzing earthquake information throughout completely different areas.
- Distant Sensing Information: Satellite tv for pc imagery and aerial images can be utilized at the side of earthquake information to determine potential fault traces, floor ruptures, and different geological options associated to earthquake exercise. Combining these datasets can refine our understanding of the boundaries and geological constructions concerned in earthquake occurrences.
- Statistical Evaluation Software program: Software program like R and Python supply instruments for spatial autocorrelation evaluation, cluster detection, and different statistical methods helpful for figuring out patterns in earthquake information. These instruments are helpful for modeling boundary delineation.
Integrating Earthquake Information with Different Information Sources
Earthquake information alone usually offers an incomplete image of tectonic plate boundaries. Integrating this information with different geological and geophysical info is essential for a extra complete and correct understanding. By combining a number of datasets, researchers can acquire a deeper perception into the complicated processes shaping these dynamic areas.
Advantages of Multi-Supply Integration
Combining earthquake information with different datasets enhances the decision and reliability of boundary fashions. This integration permits for a extra holistic view of the geological processes, which considerably improves the accuracy of fashions in comparison with utilizing earthquake information alone. The inclusion of a number of information sorts offers a richer context, resulting in extra sturdy and reliable outcomes. As an illustration, combining seismic information with GPS measurements offers a extra refined image of plate movement and deformation, thus permitting for higher predictions of future earthquake exercise.
Integrating with Geological Surveys
Geological surveys present priceless details about the lithology, construction, and composition of the Earth’s crust. Combining earthquake information with geological survey information permits for a extra full understanding of the connection between tectonic stresses, rock sorts, and earthquake prevalence. For instance, the presence of particular rock formations or fault constructions, recognized by way of geological surveys, may also help interpret the patterns noticed in earthquake information.
Integrating with GPS Information
GPS information tracks the exact motion of tectonic plates. Integrating GPS information with earthquake information permits for the identification of energetic fault zones and the quantification of pressure accumulation. By combining the places of earthquakes with the measured plate actions, scientists can higher perceive the distribution of stress throughout the Earth’s crust and doubtlessly enhance forecasts for future seismic exercise.
This mixed method provides a clearer image of ongoing tectonic processes.
Integrating with Different Geophysical Observations
Different geophysical observations, resembling gravity and magnetic information, can present insights into the subsurface construction and composition of the Earth. By combining earthquake information with these geophysical measurements, researchers can construct a extra detailed 3D mannequin of the area, serving to to refine the understanding of the geological processes at play. Gravity anomalies, as an illustration, may also help find subsurface constructions associated to fault zones, and these findings may be built-in with earthquake information to strengthen the evaluation.
Process for Information Integration
The method of mixing earthquake information with different datasets is iterative and includes a number of steps.
- Information Assortment and Standardization: Gathering and making ready information from numerous sources, guaranteeing compatibility when it comes to spatial reference methods, items, and codecs. This step is crucial to keep away from errors and make sure that information from completely different sources may be successfully mixed.
- Information Validation and High quality Management: Evaluating the accuracy and reliability of the info from every supply. Figuring out and addressing potential errors or inconsistencies is significant for producing dependable fashions. That is crucial to keep away from biased or deceptive outcomes.
- Spatial Alignment and Interpolation: Guaranteeing that the info from completely different sources are aligned spatially. If mandatory, use interpolation methods to fill in gaps or to realize constant spatial decision. Cautious consideration is required when selecting acceptable interpolation strategies to keep away from introducing inaccuracies.
- Information Fusion and Modeling: Combining the processed datasets to create a unified mannequin of the tectonic boundary. Numerous statistical and geospatial modeling methods may be utilized to the built-in information to realize a holistic understanding.
- Interpretation and Validation: Analyzing the outcomes to achieve insights into the geological processes and tectonic boundary traits. Comparability of outcomes with present geological data, together with beforehand revealed research, is essential.
Evaluating the Accuracy and Reliability of Fashions
Assessing the accuracy and reliability of boundary fashions derived from earthquake information is essential for his or her sensible software. A strong analysis course of ensures that the fashions precisely mirror real-world geological options and may be trusted for numerous downstream purposes, resembling hazard evaluation and useful resource exploration. This includes extra than simply figuring out boundaries; it necessitates quantifying the mannequin’s confidence and potential errors.
Validation Datasets and Metrics, Tips on how to use earthquake information to mannequin boundaries
Validation datasets play a pivotal position in evaluating mannequin efficiency. These datasets, unbiased of the coaching information, present an unbiased measure of how properly the mannequin generalizes to unseen information. A standard method includes splitting the accessible information into coaching and validation units. The mannequin is skilled on the coaching set and its efficiency is assessed on the validation set utilizing acceptable metrics.
Selecting acceptable metrics is paramount to evaluating mannequin accuracy.
Error Evaluation
Error evaluation offers insights into the mannequin’s limitations and potential sources of errors. Analyzing the residuals, or variations between predicted and precise boundary places, reveals patterns within the mannequin’s inaccuracies. Figuring out systematic biases or spatial patterns within the errors is crucial for refining the mannequin. This iterative strategy of evaluating, analyzing errors, and refining the mannequin is key to attaining correct boundary delineations.
Assessing Mannequin Reliability
The reliability of boundary fashions will depend on a number of components, together with the standard and amount of earthquake information, the chosen modeling method, and the complexity of the geological setting. A mannequin skilled on sparse or noisy information could produce unreliable outcomes. Equally, a complicated mannequin utilized to a fancy geological construction could yield boundaries which are much less exact than easier fashions in easier areas.
Contemplating these components, alongside the error evaluation, permits for a extra complete evaluation of the mannequin’s reliability.
Validation Metrics
Evaluating mannequin efficiency requires quantifying the accuracy of the anticipated boundaries. Numerous metrics are employed for this goal, every capturing a particular side of the mannequin’s accuracy.
Metric | Method | Description | Interpretation |
---|---|---|---|
Root Imply Squared Error (RMSE) | √[∑(Observed – Predicted)² / n] | Measures the typical distinction between noticed and predicted values. | Decrease values point out higher accuracy. A RMSE of 0 implies an ideal match. |
Imply Absolute Error (MAE) | ∑|Noticed – Predicted| / n | Measures the typical absolute distinction between noticed and predicted values. | Decrease values point out higher accuracy. A MAE of 0 implies an ideal match. |
Accuracy | (Right Predictions / Whole Predictions) – 100 | Proportion of accurately labeled situations. | Increased values point out higher accuracy. 100% accuracy signifies an ideal match. |
Precision | (True Positives / (True Positives + False Positives)) – 100 | Proportion of accurately predicted optimistic situations amongst all predicted optimistic situations. | Increased values point out higher precision in figuring out optimistic situations. |
Ending Remarks: How To Use Earthquake Information To Mannequin Boundaries
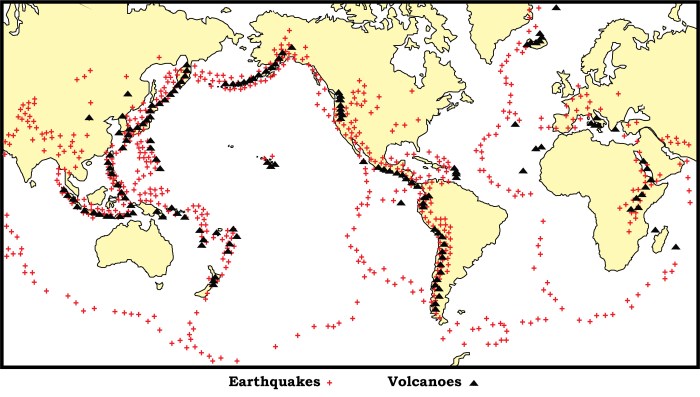
In conclusion, using earthquake information to mannequin boundaries provides a robust method to understanding plate tectonics. By meticulously processing information, using subtle modeling methods, and integrating numerous information sources, a complete and dependable mannequin may be developed. This course of allows the prediction of seismic exercise and the identification of boundaries, offering crucial insights into the dynamic nature of the Earth’s crust.
The efficient communication of those outcomes is crucial for additional analysis and public consciousness.
Important Questionnaire
What are the frequent information high quality points in earthquake datasets?
Earthquake datasets usually undergo from points resembling inconsistent information codecs, lacking location information, various magnitudes, and inaccuracies in reporting depth and focal mechanisms. These points necessitate cautious information preprocessing steps to make sure the reliability of the mannequin.
How can I predict future seismic exercise primarily based on earthquake information?
Statistical evaluation of earthquake clustering and distribution, coupled with geospatial modeling methods, can reveal patterns indicative of future seismic exercise. Nevertheless, predicting the exact location and magnitude of future earthquakes stays a major problem.
What are the advantages of integrating earthquake information with different geological information?
Combining earthquake information with geological surveys, GPS information, and geophysical observations permits for a extra holistic understanding of tectonic plate boundaries. Integrating numerous datasets improves the mannequin’s accuracy and offers a extra complete image of the area’s geological historical past and dynamics.
What are some frequent validation metrics used to guage earthquake boundary fashions?
Widespread validation metrics embody precision, recall, F1-score, and root imply squared error (RMSE). These metrics quantify the mannequin’s accuracy and skill to accurately determine boundaries in comparison with recognized boundaries or geological options.